The Kathmandu Durbar Square holds the palaces of the Malla and Shah kings who ruled over the city. Along with these palaces, the square surrounds quadrangles revealing courtyards and temples.
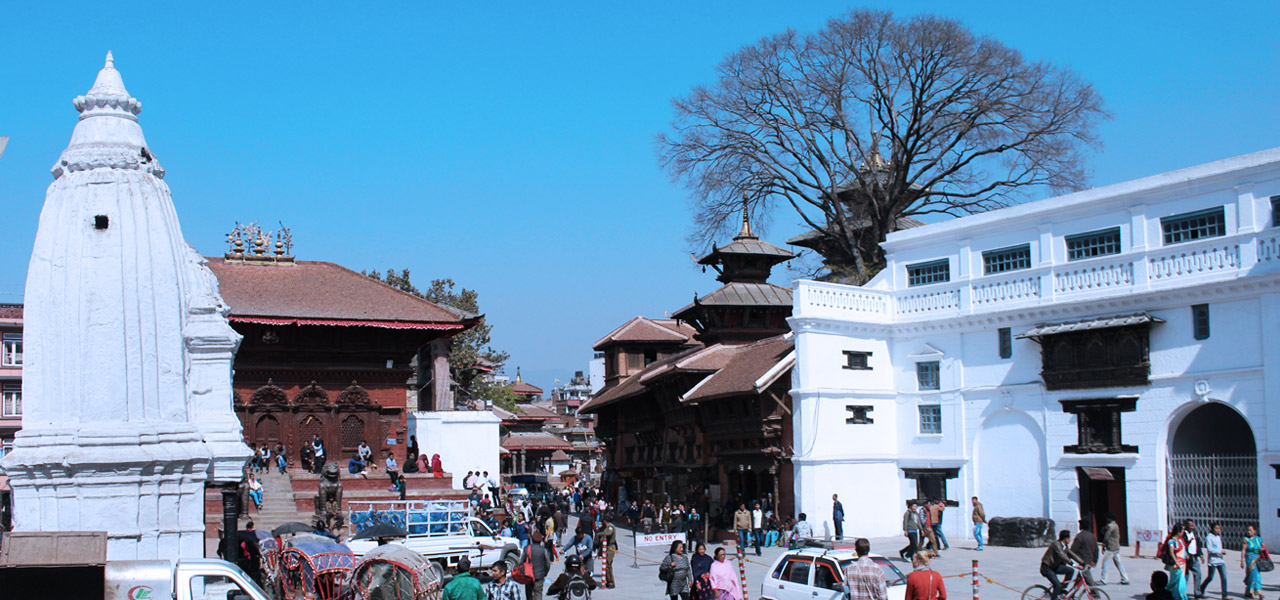
The Kathmandu Durbar Square holds the palaces of the Malla and Shah kings who ruled over the city. Along with these palaces, the square surrounds quadrangles revealing courtyards and temples.
Situated western part in the outskirts of the Kathmandu valley, Seto Gumba also known as Druk Amitabh Mountain or White Monastery, is one of the most popular Buddhist monasteries of Nepal.
Nagarkot is renowned for its sunrise view of the Himalaya including Mount Everest as well as other snow-capped peaks of the Himalayan range of eastern Nepal.
It is Simple, Clean and Responsive WordPress Theme which automatically adapts to the screen's size.
Time series analysis, a foundational component of data science, focuses on examining data points recorded in sequence, offering valuable insights across various fields like economics and meteorology. This method is essential for predicting future trends based on historical data, allowing us to extract meaningful statistics, identify patterns, and make forecasts. Key concepts within this domain include trend analysis, which helps us spot long-term trends, seasonality for identifying patterns that repeat regularly, noise separation to isolate random variations, and stationarity, assuming consistent statistical properties over time.
Techniques like descriptive analysis provide visual insights, moving averages help smooth short-term fluctuations and emphasize longer-term trends, and ARIMA models aid in forecasting. Time series analysis plays a vital role in predicting market trends, improving weather forecasts, and supporting strategic business planning. As the field evolves, machine learning methods such as Random Forests and Neural Networks are increasingly integrated, offering robust solutions for complex time series forecasting challenges.
In today’s class, we delved into the fascinating field of time series analysis, which is a branch of advanced statistics that helps us understand how phenomena evolve over time. We explored the mysteries hidden within sequences of data points and learned how to predict future trends by using past data. We also discussed essential tools like moving averages and autoregressive models, which are like magical techniques that aid in understanding how numerical values change over time.
Recognizing patterns in things like weather patterns or stock market trends is just as important in time series analysis as the mathematical aspects. Our ability to spot trends, seasonal variations, and irregularities within data is incredibly valuable for making informed decisions. This skill can be seen as a superpower in the world of data science because it empowers us to forecast and plan for the future using historical data. Today’s lesson went beyond mathematics and highlighted the practical application of numerical insights in understanding the world around us.
Logistic regression is a statistical method specifically designed for binary classification tasks, where it predicts the likelihood of an input belonging to one of two classes. It accomplishes this by using the logistic function to transform the result of a linear equation into a probability range spanning from 0 to 1. Logistic regression finds applications across various domains such as healthcare, marketing, and finance. It is used for purposes like predicting diseases, analyzing customer churn, and assessing credit risk.
The core concept of logistic regression revolves around assuming a linear relationship between the independent variables and the natural logarithm of the odds of the dependent variable. During model training, the goal is to determine the optimal coefficients, typically achieved through methods like Maximum Likelihood Estimation. The decision boundary generated by the model separates instances into their respective classes, and common performance metrics include accuracy, precision, recall, and the ROC curve.
Logistic regression’s appeal lies in its simplicity, interpretability, and effectiveness when dealing with data that can be linearly separated. These qualities have made it a widely used and enduringly popular technique in the realm of predictive modeling.
In summary, to comprehensively understand police shootings, we can analyze victim demographics, map incident locations, study temporal trends, assess police training and policies, gather community perspectives, and conduct legal and policy analysis. These dimensions provide a holistic view of the complex factors contributing to this issue. Beyond age, we can delve into the demographics of the victims, including gender, ethnicity, and socioeconomic factors. This can help us identify disparities and potential biases in police shootings
Today, I delved into the relationship between indicators of mental health issues and race in cases of police shootings. We’re utilizing a tool called facet grids in the R programming language to explore this connection. Our primary objective is to uncover any patterns or correlations that can provide insights into the complex interplay between mental health and interactions with the police.
The visual representations we generated offer a detailed perspective on how mental health and race may intersect within the context of police shootings. We will examine these visual grids closely and discuss the implications of our findings. These discoveries have the potential to inform public discussions and even influence policies concerning the handling of mental health issues during encounters with law enforcement. Join us as we navigate through these visual insights and engage in a dialogue about their significance.
K-means: This method groups data into ‘k’ number of clusters by reducing the distance between data points and the center of their assigned cluster. The ‘means’ in the name refers to averaging the data points to find the center of the cluster. It’s good for spherical-shaped clusters.
K-medoids: Similar to K-means, but instead of using the mean, it uses actual data points as the center of the cluster, known as medoids. This method is more robust to noise and outliers compared to K-means because medoids are less influenced by extreme values.
I began closely examining the data and searching for correlations between various factors. By using numerical statistics, I generated preliminary hypotheses about how certain elements such as signs of mental illness, threat levels, and attempts to flee might influence the outcomes of police interactions. These initial ideas serve as a foundation for conducting more in-depth analyses in the upcoming weeks.
It’s akin to constructing a roadmap to comprehend the intricate relationships among different aspects of these incidents. As we uncover more connections and patterns, it aids us in assembling a clearer picture of the dynamics at play in police encounters. The overarching goal is to explore and make sense of the data, allowing us to gain deeper insights into the factors that shape the outcomes of these situations.
As its clear there are casualities in the age group of teens, it’s true that half of the individuals fatally shot by the police are White, there’s a significant disparity in the rate at which Black Americans and Hispanic Americans are shot. Black Americans make up approximately 14 percent of the U.S. population but experience police shootings at a rate more than double that of White Americans. Similarly, Hispanic Americans also face a disproportionate rate of police shootings.It requires further study to get a clear picture
This week, our focus was on logistic regression, a statistical method used for analyzing the relationships between variables, particularly when the outcome of interest is binary or categorical. In other words, we were looking at situations where we want to predict whether something will happen or not, based on various factors.
During our discussions, we likely covered the fundamentals of logistic regression, including the logistic function that transforms linear combinations of variables into probabilities. This technique is commonly used in predictive modeling when dealing with outcomes like “yes” or “no,” “success” or “failure,” or “positive” or “negative.”
In addition to the theoretical aspects, it seems we also put the logistic regression into practice by performing some charting on the data variables. This might involve creating visual representations like scatter plots, bar charts, or line graphs to explore how the variables relate to each other and how they might influence the binary outcome we are interested in.
In essence, this week’s focus on logistic regression and data visualization equips us with the analytical tools needed to make sense of data, uncover hidden trends, and use this knowledge to make informed decisions. It’s a fundamental step in the process of applying statistical methods to address practical problems and contributes to our ability to navigate complex real-world scenarios with confidence.